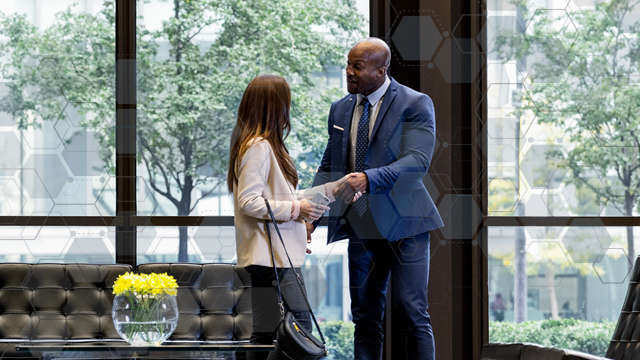
What’s Next for AI in Financial Services in 2020? The 5 Top Trends
Follow Pascal Belaud on LinkedIn
Right now, banking, capital markets, and insurance businesses are finding new uses for artificial intelligence. AI is helping to transform and keep ahead of a number of critical vectors, including customer experience, regulatory compliance, new markets and business models, and security. It’s no longer a “nice to have” innovation technology, it’s becoming a CX-level imperative. In this very moment, financial service organizations are making space for AI through financial resourcing, culture adaptation, and workforce skilling.
To get ready for what’s coming in 2020 for AI in the financial services industry, let’s look at the top emerging trends:
Trend 1: Enterprise adoption of the Data Science Lifecycle Process
One enormous problem we’ve seen, particularly in financial services, is the challenge of achieving ROI on data science. Eighty-seven percent of data science projects fail, mostly because of a misalignment on process.
One of the points of misalignment is the shape of the process. IT and business departments expect the data science process to be linear, when it really isn’t. As a result, data scientists try to conform to a linear process and leverage existing tools, and that can slow things down. Many data scientists aren’t even ready to get down to brass tacks until three months into their role. Since IT doesn’t have a great understanding of data science, a lot of the work doesn’t make it into production. When there is no way to measure the impact of the data scientist’s work, it can be terribly frustrating to the scientist. However, because data scientists are such a hot commodity, there’s always another, better paying job for them to leap to. In fact, the average data scientist stays at a company for only 10 months, unfortunately bringing all of their learnings with them. This leaves the company out a good amount of money for very little reward in the end.
Microsoft has recently partnered with GitHub to create some brand-new programming to layer the linear DevOps model over the non-linear data science model, allowing for a smoother process and greater democratization of valuable kernels of learning along the way. Stay tuned to this blog; we’ll be rolling out more information on this new solution as it comes. In the meantime, if you are interested in knowing more about this topic, feel free to get in touch: mailto:dslp@microsoft.com
Trend 2: AI is creating the ‘superworker’
Initially, we in the tech industry thought that AI was going to automate a big swath of jobs, but that’s actually not what we’re seeing emerge today. Instead, we’re seeing companies working on augmenting the human worker with a suite of technology that makes them a “superworker,” giving one worker the power of multiple workers.
Why you don’t want to take the human out of the “Mech Suit” just yet: AI models are really good at predicting what was going to happen yesterday, so you need to have humans engaged to be able to give the kind of insights that only humans can provide. Things change; the ground-level truth is going to shift. If you give humans the ability to interact with those models and input the ground-level information back into the systems, you go from a static, limited data model, to a more accurate, living model.
While much of the training of the human workforce will be on the job, organizations can help employees develop their skillsets through directing them to the Microsoft AI Business School, a no-cost, holistic resource and hands-on learning to get them more comfortable in the business world of AI.
Trend 3: The rise of living models
Static models are the way of the past. In a static model, the AI might passively predict that the next best product to sell to Audience Y is Product A. However, the human salesperson on the ground level might disagree with this model and leverage their expertise to recommend selling Product B. Through inputting the expertise back into the model, workers become involved in retraining the AI and creating a living model.
Workers can help train models through simple interaction, which could be a basic, “thumbs up,” or “thumbs down,” or checking a box; or through explicit interaction, which involves employees dictating to the model exactly what information it got wrong, and what it got right.
Let’s put this into practice: an insurance worker reviewing claims can leverage an AI model to help predict if a claim is fraudulent. The model might flag a claim with 80 percent certainty that the claim is fraudulent, however, the human with years of experience handling these kinds of claims might review the model’s findings and determine that, in fact, the claim is not fraud. The human worker feeds their knowledge back into the model, helping train the model for greater accuracy.
Trend 4: The birth of Accountable AI
A huge objective in financial services is to be able to build models that are free of unwanted bias. For example, you don’t want a married couple with the same financial standing to apply for a credit card, only to discover that the wife has been denied based on apparent gender bias in the model. You need to be able to leave bias variables out with accuracy, which is why we need Accountable AI.
Financial services has been held back by regulations from using more accurate “black box” models that have lacked transparency in favor of models that are less accurate but with high explain-ability. Unfortunately, less accuracy means they’re leaving money on the table. Microsoft has invented Explainable Boosting Machines (EBMs) that can deliver greater accuracy as well as transparency, allowing companies to measure the exact impact of each variable in a model and manually edit for biases behind the scenes.
Visit the GitHub link to get more information on, or to try the new explainable algorithm: https://github.com/interpretml/interpret
Trend 5: It’s time to reskill the workforce
A lot of organizations are putting considerable thought into reskilling their workforce. With data models being fed back by humans, they have to make sure the workforce has an understanding of how machine learning works. They also need to train existing skilled workers to do their job slightly differently or to take on different roles.
One NGO, NPower is working to reskill the workforce through helping young adults and veterans obtain skills in IT and placing them in internships in industries like finance. Microsoft is creating an AI curriculum for the program to teach students how to be a liaison between IT and business and data science and machine learning. Eventually, we plan to deploy this curriculum across universities so that we can train as many people as possible on AI.
Stay tuned to this blog for more thoughts on what’s next for AI in the financial services industry. More usable information and helpful insights are coming your way.