New – HAMS Automated License Testing Dashboard is Live!
Context
Road safety is a major public health issue, accounting for an estimated 1.35 million fatalities, and many more injuries, the world over, each year, placing it among the top 10 causes of death. Middle-income and particularly low-income countries bear a disproportionate burden of road accidents and fatalities. For instance, the estimates of road fatalities in India range from one every 4 minutes to almost a quarter of a million, or 20% of the world’s total, each year. Besides the heavy human cost, road accidents also impose a significant economic cost. So it is no surprise that the problem has attracted attention at the highest levels of the government, including from Prime Minister Modi himself during a radio address in 2015.
The major factors impacting safety — vehicles, roads, and drivers — see little or no ongoing monitoring today, especially in countries such as India. It is our thesis that improving road conditions, vehicle health and, most importantly, driver discipline would help boost road safety. Indeed, among the leading causes of road accidents are such factors as speeding, drunk driving, and driver distractions, all of which can be mitigated through better driver discipline.
HAMS overview
In the Harnessing AutoMobiles for Safety, or HAMS, project, we use low-cost sensing devices to construct a virtual harness for vehicles. The goal is to monitor the state of the driver and how the vehicle is being driven in the context of a road environment that the vehicle is in. We believe that effective monitoring leading to actionable feedback is key to promoting road safety.
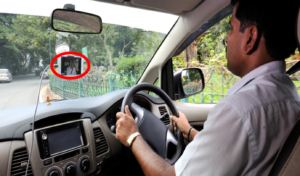
Smartphone setup in HAMS
The sensing device employed in HAMS is an off-the-shelf smartphone. The smartphone is mounted on the windshield, with its front camera facing the driver and the rear camera looking out to the front. The key to the operation of HAMS is the use of multiple sensors simultaneously. For example, when a sharp braking event is detected (using the smartphone’s accelerometer), the distance to the vehicle in front is checked (using the rear camera), along with indications of driver distraction or fatigue (using the front camera). Such sensing and detection in tandem helps provide a holistic and accurate picture of how the vehicle is being driven, enabling appropriate feedback to then be generated.
The research challenges we address in HAMS pertain to effective detection and monitoring in challenging settings. Some of these challenges arises because HAMS is retrofitted onto legacy vehicles, and so it must contend with variation in vehicle configuration, driver seating, and even the smartphone mounting. Other challenges arise because of our goal to be broadly applicable, including in regions where we cannot count on well-marked, fixed-width lanes, for instance, to perform vehicle ranging. We also address the challenge of efficient operation on a smartphone with modest resources, for instance, by combining accurate deep learning models with less expensive traditional computer vision techniques.
As part of the project, we have also explored several use cases for HAMS. One of the earliest we prototyped was a fleet management dashboard, which allowed a supervisor to view safety-related incidents of interest offline. We have also piloted HAMS in the context of driver training, in collaboration with the Institute of Driving and Traffic Research (IDTR), run by Maruti-Suzuki, the largest passenger car manufacturer in India.
More recently, we have been working with several State Transport Departments on using HAMS to automate the driver license test. See Automated License Testing for more details.
Visit our other tabs at the top of this page for more information.
People
Akshay Nambi
Principal Researcher
Venkat Padmanabhan
Managing Director, Microsoft Research India