Set-Sequence-Graph: A Multi-View Approach Towards Exploiting Reviews for Recommendation
- Jingyue Gao ,
- Yang Lin ,
- Yasha Wang ,
- Xiting Wang ,
- Zhao Yang ,
- Yuanduo He ,
- Xu Chu
ACM International Conference on Information and Knowledge Management (CIKM) |
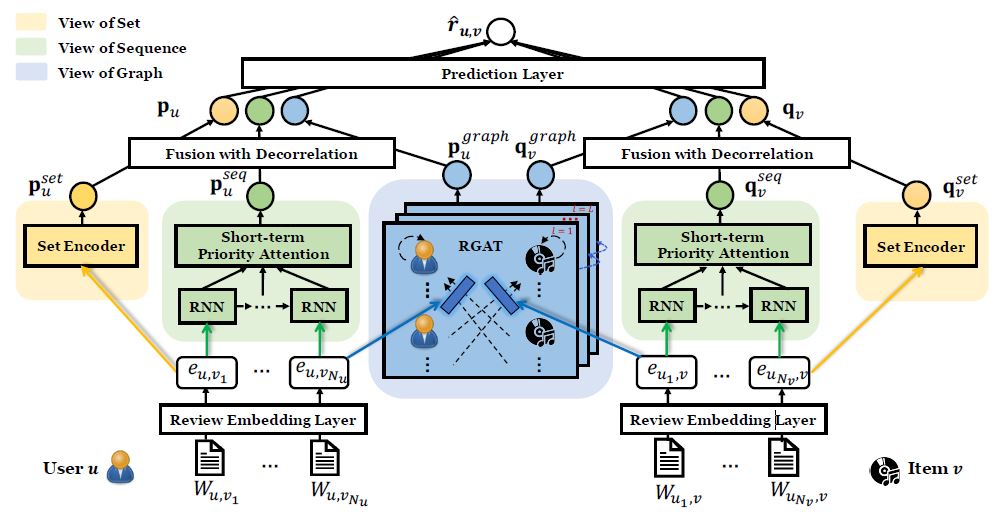
Existing review-based recommendation models mainly learn long-term user and item representations from a set of reviews. Due to the ignorance of rich side information of reviews, these models suffer from two drawbacks: 1) they fail to capture short-term changes of user preferences and item features reflected in reviews and 2) they cannot accurately model high-order user-item collaborative signals from reviews. To overcome these limitations, we propose a multi-view approach named Set-Sequence-Graph (SSG), to augment existing single-view (i.e., view of set) methods by introducing two additional views of exploiting reviews: sequence and graph. In particular, with reviews organized in forms of set, sequence, and graph respectively, we design a three-way encoder architecture that jointly captures long-term (set), short-term (sequence), and collaborative (graph) features of users and items for recommendation. For the sequence encoder, we propose a short-term priority attention network that explicitly takes the order and personalized time intervals of reviews into consideration. For the graph encoder, we design a novel review-aware graph attention network to model high-order multi-aspect relations in the user-item graph. To combat the potential redundancy in captured features, our fusion module employs a cross-view decorrelation mechanism to encourage diverse representations from multiple views for integration. Experiments on public datasets demonstrate that SSG significantly outperforms state-of-the-art methods.