Personas in agriculture
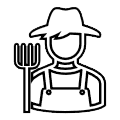
Farmer
Decision making for seeds, purchases, and management
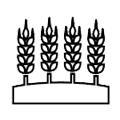
Input provider / Agronomist
Communicate with farmer & advisories, input purchase decisions
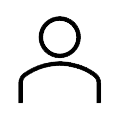
Consumer
Nutrition and organic, sustainable produce
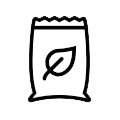
CPG/Retailer
Purchasing decisions, sustainability
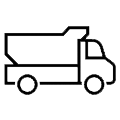
Food manufacturing / Supply chain
Coordinate with farmers on prices and food standards
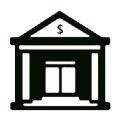
Banker
Communicate with farmers about loans and insurance
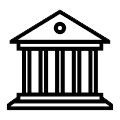
Policy maker
Make policy documents accessible to farmers
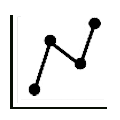
Researcher / Data scientist
AI workflow automation
Customizing LLMs
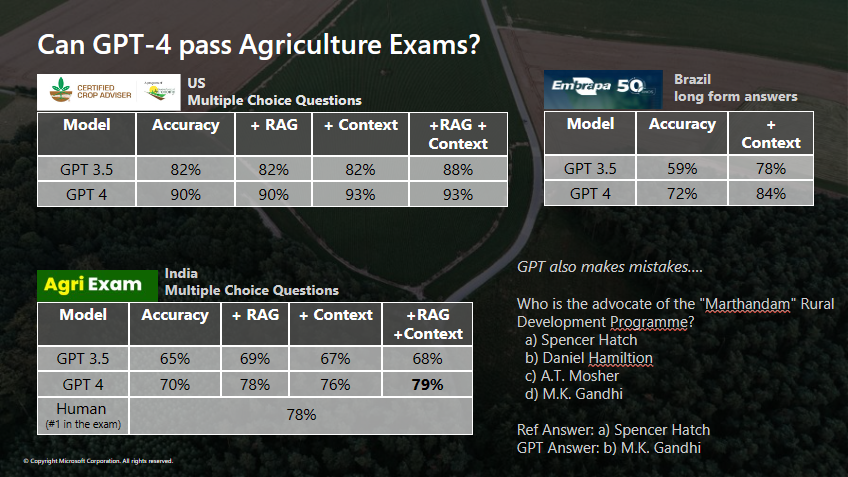
Agronomist exam
One of the challenges in the agriculture sector is the lack of qualified agronomists who can provide expert advice on crop production and protection. To address this gap, Microsoft Research conducted a study that evaluates the performance of LLMs like GPT-4 in answering agriculture-related questions from certification exams in Brazil, India, and the USA. The results show that GPT-4 can achieve high scores on these exams, demonstrating its ability to understand and generate domain-specific knowledge. The study also suggests that LLMs can be used as an agronomist assistant, providing guidance and feedback to farmers and students.
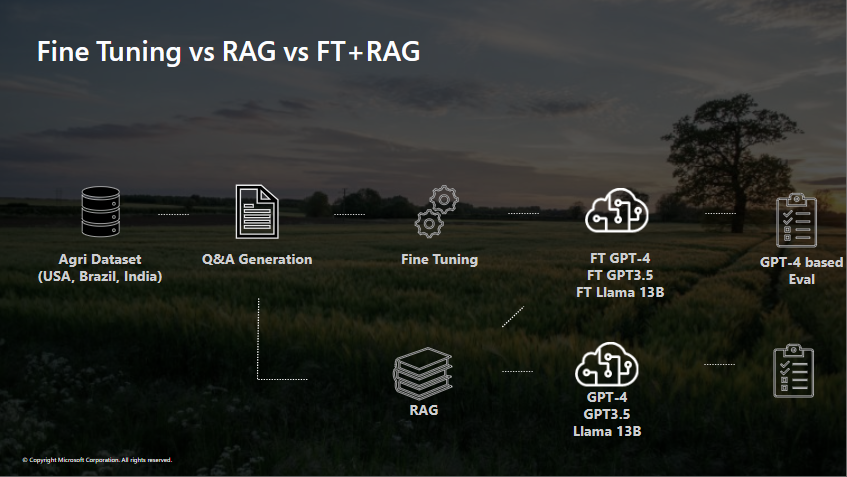
RAG vs Fine-Tuning: A case study on agriculture
Another challenge in applying LLMs to agriculture is the scarcity of domain-specific data that can enhance the models’ performance and accuracy. To overcome this limitation, Microsoft Research explored two techniques to augment LLMs with additional localized data, focusing on agriculture: fine-tuning and Retrieval-Augmented Generation (RAG). Fine-tuning involves training the LLMs on a subset of data that is relevant to the target domain, while RAG involves retrieving relevant documents from a large corpus and using them as context for the LLMs. The study compares and contrasts these techniques on multiple popular LLMs, including Llama2-13B, GPT-3.5, and GPT-4, and evaluates their performance on various tasks, such as question answering, text summarization, and text generation. The study also discusses the implications of these techniques on the LLMs’ generalization and robustness.
Small Language Models and Edge
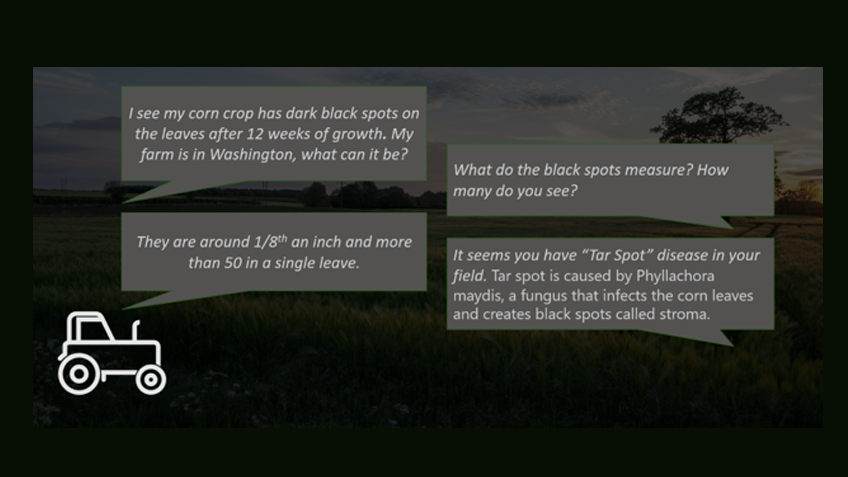
A third challenge in leveraging LLMs for agriculture is the dependency on internet connectivity and cloud computing, which may not be available or reliable in remote or rural areas. To address this challenge, Microsoft Research has been exploring the deployment of LLMs on edge devices, such as smartphones, tablets, or laptops, that can allow for faster processing and response times, as well as the ability to operate in environments with limited or no internet connectivity. One such example is a farmer with no connectivity running LLM on a tractor or sprayer and using it to answer queries based on the manuals instead of flipping through hundreds of pages. This is just an example of the work that Microsoft Research has been doing to enable LLMs on the Edge.
Multi-modal GenAI
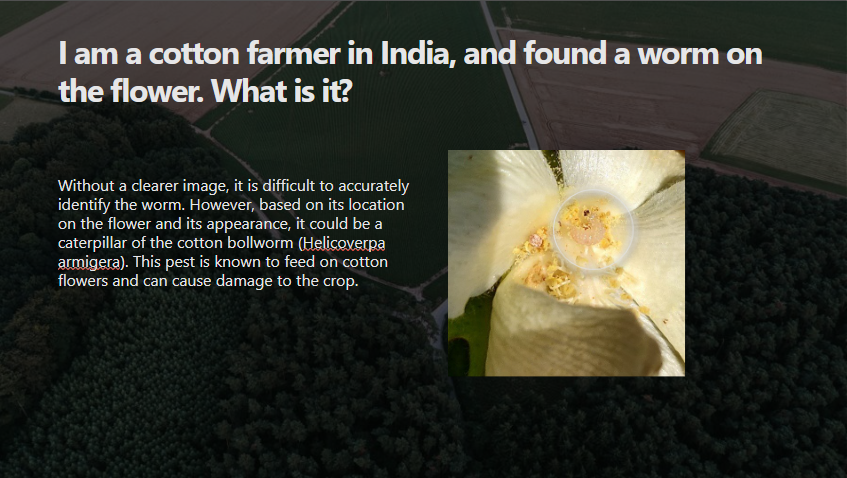
A fourth challenge in applying LLMs to agriculture is the multi-modality of data that can enhance the models’ performance and accuracy which involves the integration of multiple types of data, such as text, images, video, satellite and audio to improve AI models’ understanding and decision-making. This is particularly useful in the agriculture industry, where data can come in various forms, such as weather reports, satellite images of crops, and audio recordings of livestock. By integrating these different types of data, multi-modal LLMs can provide more accurate and comprehensive insights and recommendations for farmers and agronomists. For example, a multi-modal LLM could analyze satellite images of a field to identify areas of drought or pest infestation, and then generate a report with recommendations for irrigation or pesticide application. This is just one example of the potential applications of multi-modal LLMs in agriculture.