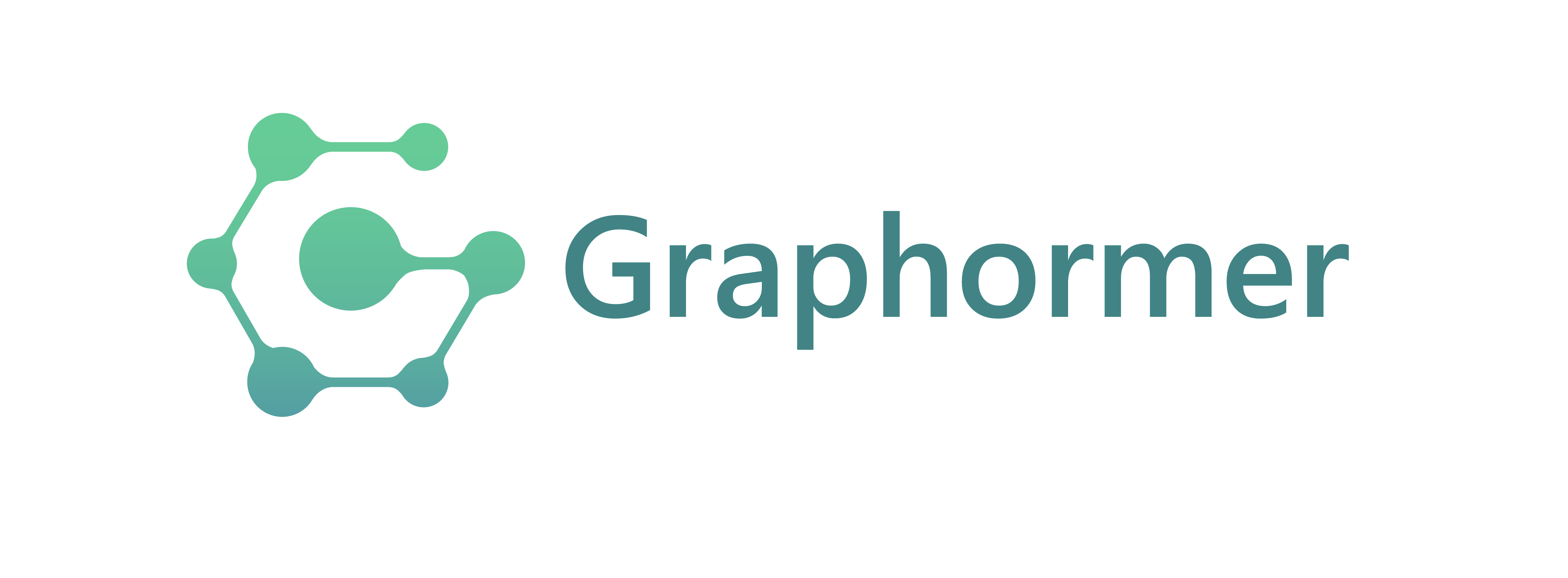
Graphormer is a deep learning package that allows researchers and developers to train custom models for molecule modeling tasks. It aims to accelerate the research and application in AI for molecule science, such as material discovery, drug discovery, etc. Now it supports various molecule simulation tasks, e.g., molecular dynamics and property prediction. In the near future, it will further support important applications in molecule science, such as reaction prediction, retrosynthesis, molecule generation, large-scale molecule (polymer, protein) modeling, drug-protein interaction (DPI), and so on.
Graphormer is initially described in NeurIPS 2021 paper Do Transformer Really Perform Badly for Graph Representation Learning? The proposed Graphormer is the first deep learning model built upon a standard Transformer that greatly outperforms all conventional graph neural networks on graph-level prediction tasks.
Graphormer won first place in the KDD Cup – OGB-LSC (opens in new tab) quantum chemistry track, which aims to use AI to predict the quantum properties of more than 3.8 million molecules. This competition attracts more than 500 teams to participate, including DeepMind and other big companies. Recently, Graphormer won the Open Catalyst Challenge, a molecule dynamics competition that aims to model the catalyst-absorbate reaction system by AI, and has more than 0.66 million catalyst-absorbate relaxation systems (144 million structure-energy frames) simulated by density functional theory (DFT) software. Winning the OC challenge demonstrates that Graphormer could largely benefit the development of new AI technology for material discovery, and also other important areas.
Advanced pre-trained versions of Graphormer are available exclusively on Azure Quantum Elements (opens in new tab).
People
Chang Liu
Senior Researcher
Tie-Yan Liu
Distinguished Scientist, Microsoft Research AI for Science
Yu Shi
Researcher