Navigation Turing Test (NTT): Learning to Evaluate Human-Like Navigation
- Sam Devlin ,
- Raluca Stevenson ,
- Ida Momennejad ,
- Jaroslaw Rzepecki ,
- Evelyn Zuniga ,
- Gavin Costello ,
- Guy Leroy ,
- Ali Shaw ,
- Katja Hofmann
2021 International Conference on Machine Learning |
Source code available at: https://github.com/microsoft/NTT
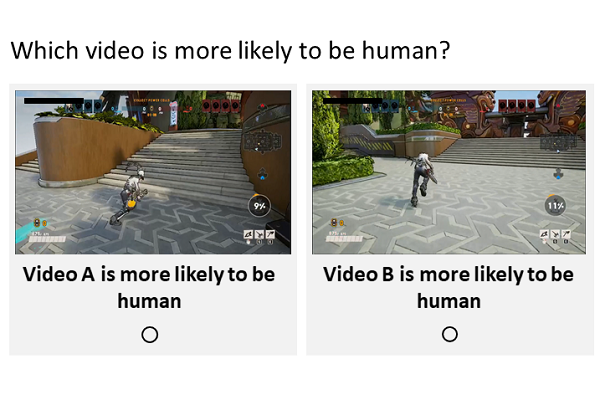
A key challenge on the path to developing agents that learn complex human-like behavior is the need to quickly and accurately quantify human-likeness. While human assessments of such behavior can be highly accurate, speed and scalability are limited. We address these limitations through a novel automated Navigation Turing Test (ANTT) that learns to predict human judgments of human-likeness. We demonstrate the effectiveness of our automated NTT on a navigation task in a complex 3D environment. We investigate six classification models to shed light on the types of architectures best suited to this task, and validate them against data collected through a human NTT. Our best models achieve high accuracy when distinguishing true human and agent behavior. At the same time, we show that predicting finer-grained human assessment of agents’ progress towards human-like behavior remains unsolved. Our work takes an important step towards agents that more effectively learn complex human-like behavior.
Publication Downloads
Navigation Turing Test (NTT): Learning to Evaluate Human-Like Navigation [Dataset]
July 9, 2021
Research talk: Evaluating human-like navigation in 3D video games
On the path to developing agents that learn complex human-like behavior, a key challenge is the need to quickly and accurately quantify human-likeness. While human assessments of such behavior can be highly accurate, speed and scalability are limited. The researchers address these limitations through a novel automated Navigation Turing Test (NTT) that learns to predict human judgments of human-likeness. They demonstrate the effectiveness of their automated NTT on a navigation task in a complex 3D environment. They investigated six classification models to shed light on the types of architectures best suited to this task, and they validated them against data collected through a human NTT. The best models achieve high accuracy when distinguishing true human and agent behavior. At the same time, the researchers show…