By John Kaiser, Writer, Microsoft Research
Microsoft Research hosted its third annual Data Science Summer School (opens in new tab) in New York City as a diverse group of undergraduate students deployed some of the latest data crunching techniques on millions of rows of anonymized data in an effort to uncover useful information.
“We’re really hoping to give them a flavor of solving a research problem that hasn’t yet been solved,” said Jake Hofman (opens in new tab), one of several Microsoft Research instructors leading the intensive eight-week hands-on course that concluded in August. Coursework (opens in new tab) for the program is freely available on Github.
Data points to tweaking incentives at Airbnb
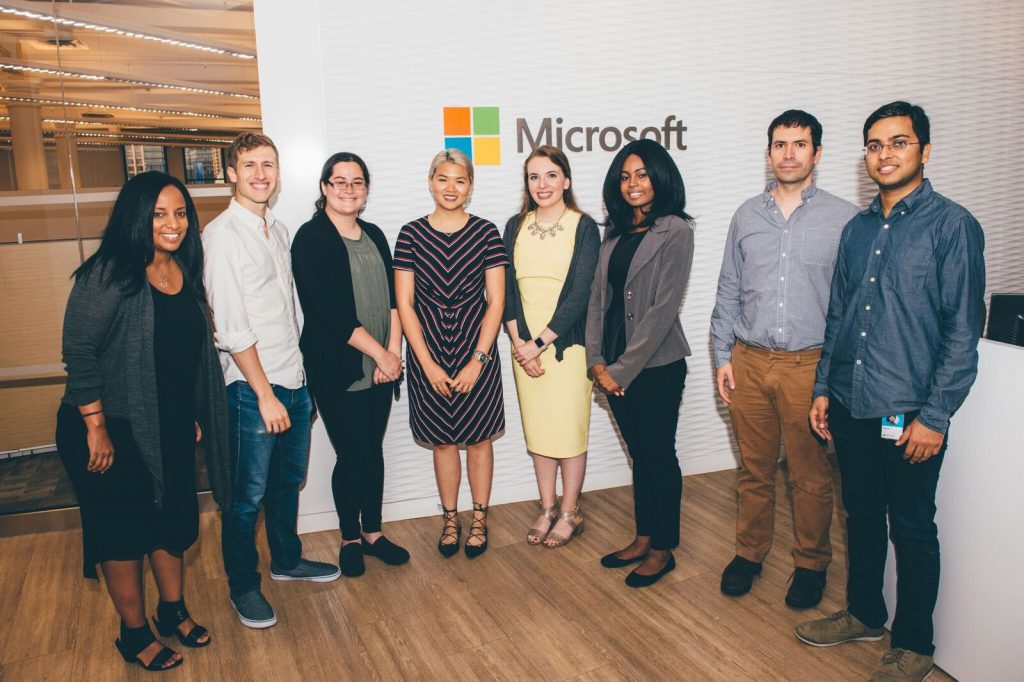
Spotlight: Blog post
AirBnB team left to right: Shawndra Hill (MSR, mentor), Chris Riederer (Columbia, teaching assistant/mentor), Erica Ram (student), Louise Lai (student), Jacqueline Curran (student), Kaciny Calixte (student), Fernando Diaz (MSR, mentor), Amit Sharma (MSR, mentor)
This year marked the first time that student-led research relied on machine learning algorithms to predict actual outcomes. In a project called “Airbnb: Predicting Loyalty (opens in new tab),” the students tapped decision tree learning techniques — “using decision trees to find patterns in the given data to predict on unseen data.” Most importantly, they were able to pinpoint how the company might tweak specific factors to encourage guests to book another stay or incentivize hosts to open up their home another time.
Students looked for patterns indicating a higher or lower probability of being a repeat customer.
“How does host loyalty interplay with guest loyalty?” asked summer school student Louise Lai, in describing one of the primary areas of focus for the Airbnb study group. “We’re looking at that interplay as something very new and very distinct for the sharing economy.”
For the Airbnb student project, Lai was joined by Kaciny Calixte, Jacqueline Curran and Erica Ram.
Explaining how “predictive models show that reviews and interaction between hosts and guests is of great importance,” the study concluded that “Airbnb could potentially boost return-rates of first time guests by providing them with incentives to stay at highly-rated properties.”
The project relied on two datasets collected by InsideAirbnb (opens in new tab), which describes itself as an “independent, non-commercial set of tools and data” that allows anyone to “explore how Airbnb is really being used in cities around the world.”
In another sign of the maturing field of data science, this year marked the first time that student projects used pre-existing datasets without the need for modification.
“It does raise the bar for the types of questions that get asked. We’re seeing the tools improve, we’re seeing more and more interesting datasets out there.
And certainly Microsoft Azure’s point and click graphical interface makes it seem easy — if you know what to look for.
“What were’ trying to train our students on is more around what questions to ask and how to answer them,” Hofman added.
Taxi data points to carpooling to push to counter redundant trips
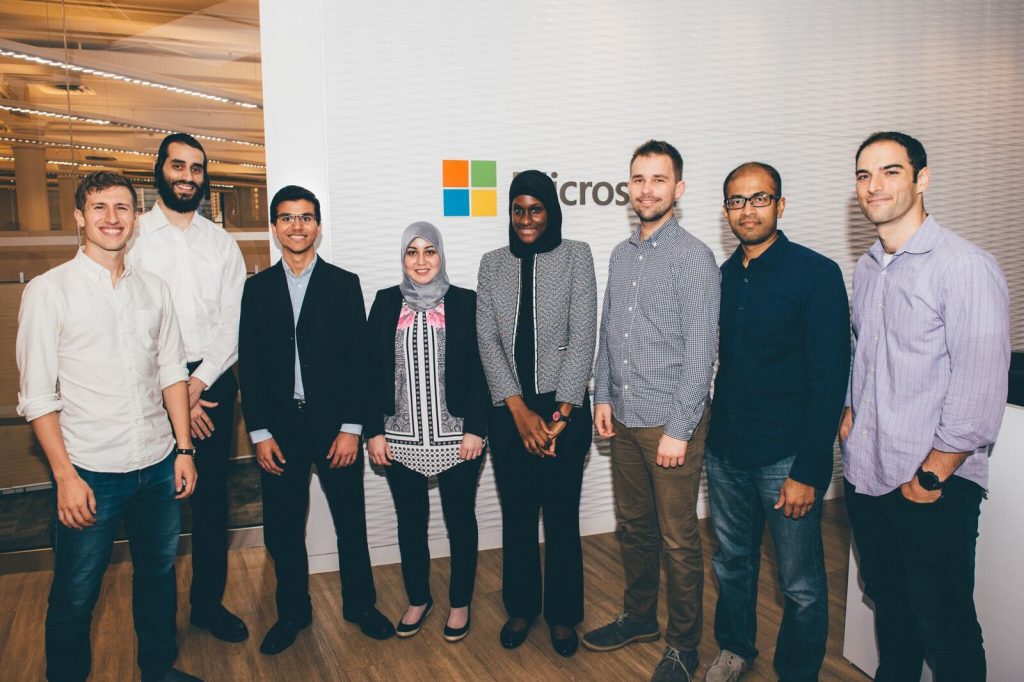
Fare Share team left to right: Chris Riederer (Columbia, teaching assistant/mentor), Abraham Neuwirth (student), Jai Punjwani (student), Fatima Chebchoub (student), Marieme Toure (student), Ashton Anderson (MSR, mentor), Sid Sen (MSR, mentor), Jake Hofman (MSR, mentor)
The other student project, “Fare Share: Flow and Efficiency in NYC’s Taxi System (opens in new tab),” tapped into what’s officially known as the “2013 Yellow Taxi Driver Set,” which contains anonymized driver IDs, trip time, distance, point of origin, destination and other information.
In the group’s final presentation, summer school student Jai Punjwani described the dataset this way: “Imagine if you have info on every single cab in New York City and you able to see where every single cab was going — who they were picking up, who they were dropping off, what they were doing afterward.”
Punjwani is now entering his junior year in computer science at Adelphi University, where he’s developed an Android app that enables students “to find each other and study at his university.” For the taxi data student project, Punjwani was joined by Abraham Neuwirth, Marieme Toure and Fatima Chebchoub.
The research project focused on a single month of data, which included more than 13 million rides, for an average of 420,000 trips per day, driven by over 32,000 different drivers.
Unlike a similar project in 2009 that yielded largely inconclusive results, this year’s study zeroed in on longer trips to specific destinations, revealing that large numbers of taxis ferried just a single passenger on various popular commute and transit routes. The study notes that on “weekday mornings around 7 a.m., there are roughly 25 redundant trips from Port Authority to Rockefeller Center that take place every five minutes for the duration of rush hour.”
The students concluded that a “taxi stand policy requiring people to wait no more than five minutes to carpool with another rider at these locations could improve the system by upwards of 5 percent, eliminating more than 650,000 trips. That translates into a potential savings to consumers of more than $8.5 million.”
It’s a good example of how data science can shine a light on efficiencies that would otherwise go unnoticed, and it shows how research could lead spur new policies.
“You could really improve the efficiency of the taxi system that could happen at almost zero cost,” Hofman noted.
There’s a reason it’s called “big data.” For their projects, students were faced with the tricky task of culling through millions of rows of data, discarding anomalies like multiple Airbnb listings or faulty geolocation taxi journey data such as an erroneous trip to Antarctica.
Microsoft Research Data Science Summer School
The program was launched in 2014 as part of a commitment to boost the diversity in computer science, encouraging “applications from women, minorities, people with disabilities and students from resource-limited colleges.” This year’s class included a woman who immigrated from Senegal and another who moved from Morocco.
In choosing applicants from more than 100 entries, Microsoft looks for candidates who have demonstrated a degree of passion around computer science from their undergraduate coursework and related activities.
Projects from earlier years drew on data from New York’s public school system, subway and fleet of shared bicycles, as well as stats compiled from ongoing police practices.
Summer 2016 Projects
Airbnb: Predicting Loyalty
Watch the talk (opens in new tab) or read the paper (opens in new tab) for more details. Source code is available on GitHub (opens in new tab).
Fare Share: Flow and Efficiency in NYC’s Taxi System
Watch the talk (opens in new tab) or read the paper (opens in new tab) for more details. Source code is available on GitHub (opens in new tab) as well as an interactive map of travel patterns across neighborhoods (opens in new tab).