By Penny Collisson, Gwen Hardiman and Trish Miner
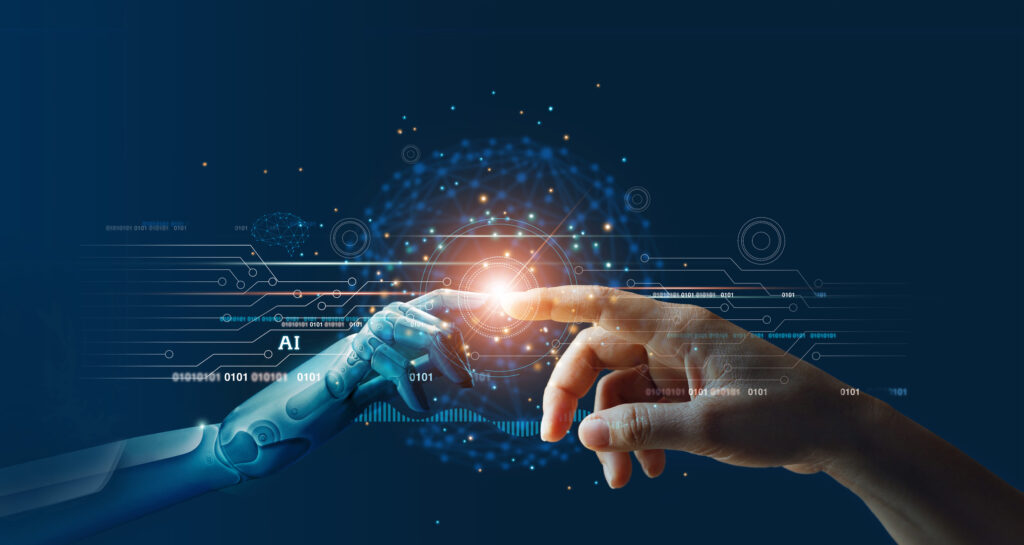
Photo credit: iStock
Pssst. Users don’t trust your AI.
It’s OK. Don’t panic. You have an opportunity to change that, and here’s how.
What we know
Despite advances in technology, users are still hesitant to fully trust the capabilities and suggestions of AI. Given the example of trusting a human or trusting AI, people will choose the human, even when the human is wrong. Perhaps surprisingly, when the human is shown to be wrong repeatedly, the person seeking suggestions will still choose the other human. Here’s how to bridge those two worlds and build trust.
Designing for trust
Designing for trust is about putting people at the center of our approach – their humanity, intents, and feelings – not technology. Empathizing with people, and obsessing over our relationship with them, must go hand in hand with leveraging the superpowers of intelligent tech. Here are three four practical things you can start doing today to design with trust in mind:
- Walk through your user’s experience as you design. Sometimes the user’s idea of how a particular feature is supposed to work differs from the designers or the engineers. That’s often because people are using your intelligent feature in the context of other things. One trick you can use when evaluating a new AI–based feature is to look at it in the context of a likely end to end user story.
- Prototype with real user data. It can be tricky to anticipate all the ways your AI might go wrong before you have an algorithm. One suggestion is to walk through your experience yourself using real user content. You can also use people’s data to simulate a wrong answer, and then get them to respond. This approach can highlight gaps you might have otherwise overlooked. Once you know the gotchas, you’re better equipped to make use of the guidance on just how to design for being wrong. Prototyping with real data helps you get ahead of being wrong.
- Ask people for feedback, then act on it. Research suggests that involving people more with their AI not only improves trust, but also allows the AI to learn from people’s experience of it. This is because it enables users to direct AI so it benefits them most and leaves them feeling in control.
- Get the data about what’s working. The next step is to ensure the experience we’re shipping, even when it is not yet the best it can be, is good enough to get the repeat usage we need to get data about what’s working. In our work with product teams, we aim to ship once we’re confident we have achieved a trustworthy experience.
Measuring trust
How do we operationalize and measure the trustworthiness of an experience before we ship? People’s feelings, thoughts, and actions reflect trust. Looking at all three dimensions helps us get a fuller picture of a human being and determine whether they trust an experience. Applying the lens of feel-think-act can help you structure your evaluations, make sense of people’s feedback, and justify your decision to move forward and ship, or iterate one more time.
To measure if an experience is trustworthy, consider how someone will feel, think, and act
- Feelings: Positive emotions contribute to habit formation and retention. When determining if you have a trustworthy experience, you want to pay particular attention to feelings that ladder up to trust. For example:
- Make sure positive feelings like feeling Confident, or Secure don’t decrease
- Make sure negative feelings like Uncertainty or Frustration don’t increase
To track these feelings, you can survey people directly, capturing feelings before they use the experience and afterwards to see if there’s a change. You can also observe feelings while people use your experience or prototype. Sighs of frustration or a relaxed, confident body posture will often reveal a truer picture than a circled number on a survey scale.
- Thoughts: Thoughts encapsulate opinions, beliefs and perceptions. In evaluating whether you have a trustworthy experience, you want to pay particular attention to thoughts on value and comprehension which are basic to trust.
- Listen for affirmations – things that sound like what you’re after. E.g., “X is useful to me”; “At first glance I understand X”.
- Listen for contraindications – things that are in opposition to what you’re after. E.g., “I’m worried X will hinder not help me”; “I don’t understand what to do here.”
While you likely won’t hear people say these statements word-for-word, writing them down in advance will help you focus on themes to listen for in feedback and reflections.
- Actions: Actions are what people do in and around your experience. We track actions – like return usage – through telemetry. Before shipping, you can get a pulse for likelihood to try and use. You can:
- Ask people directly whether they would try and return to your experience; and
- Use what you learn during evaluations to infer likelihood to use. Ask about current habits, think about how they map to your use cases, and consider whether the person had a positive, negative or neutral experience. Combine this knowledge with direct answers to control for “researcher pleasing” as people often aren’t great at predicting their own behavior.
In sum, evaluating through a lens of trust helps us put the focus on people over product. Use the feel–think-act framework to understand if you have a trustworthy experience even before shipping and help create great AI-infused experiences people will want to come back to again and again.
At Microsoft, we have a centralized approach to responsible AI, led by Microsoft’s AI, Ethics, and Effects in Engineering and Research (AETHER) Committee along with our Office of Responsible AI (ORA). Together, AETHER and ORA work closely to ensure we build responsible AI into our products and services. You can learn more about our principles at our Approach to AI webpage and find resources to help you develop AI responsibly in our Responsible AI Resource Center.
What do you think? How might these ideas enhance your development of AI? How does this resonate with your own research and experience? Tweet us your thoughts @MicrosoftRI or follow us on Facebook and join the conversation.
Penny Collisson leads a team of passionate researchers working on AI and platform capabilities across Office.
Gwen Hardiman is a Senior Design Researcher at Microsoft who led work on designing for trustworthy experiences.
Trish Miner is a Principal User Research Manager at Microsoft with a passion for creating desirable experiences through focusing on how people think, act and feel.